Task 2.1 Modeling of smart bricks.
Analytical models for smart bricks will be formulated able to describe their input (strain/damage) – output (electrical properties) response under application of mechanical loading. Smart Masonry enabling SAFEty-assessing STructures after earthquakes (SMS-SAFEST) Lumped circuit approaches and micromechanical approaches will be integrated to achieve such models (Figure 4). The developed models will be experimentally validated in small structural walls.
Task 2.2 Modeling of smart mortars.
Analytical models for smart mortar layers will be developed, linking a damage (crack crossing the mortar layer) to a local change in electrical properties, integrating micromechanical and lumped circuit approaches (Figure 4). Experimental testing on small specimens, as well as on scaled-down masonry structural components (e.g. walls and arches) with smart mortar layers, will be carried out to systematically validate the analytical models.
Task 2.3 Electromechanical and environmental characterization.
Electromechanical and environmental characterization of the new materials will be carried out, seeking analytical relations between electromechanical parameters of the developed composites, mechanical strains and environmental conditions. Mechanical characterization, including stress-strain constitutive behavior, ductility, mechanical strength and more, will also be carried out.
Task 2.4 Numerical validation.
Task 2.4 will be focused on numerically assessing the crack detection potential of smart mortar and smart bricks when embedded into simple structural models (e.g. small walls under shear loading and arches under horizontal loading) through numerical simulations. Data fusion approaches will be exploited for discriminating different failure mechanisms and assessing damage severity.
Task 2.5 Experimental tests on small-scale structural specimens.
Small scale structural components will be tested to verify the effectiveness of smart mortar layers and smart bricks and the achieved measurement accuracy. Tests will cover both elastic range of deformation as well as the ultimate limit state. Tested components will comprise diagonal compression tests on walls, as well as tests on small structural assemblies, such as scaled-down arches and vaults, under both vertical and lateral loading.
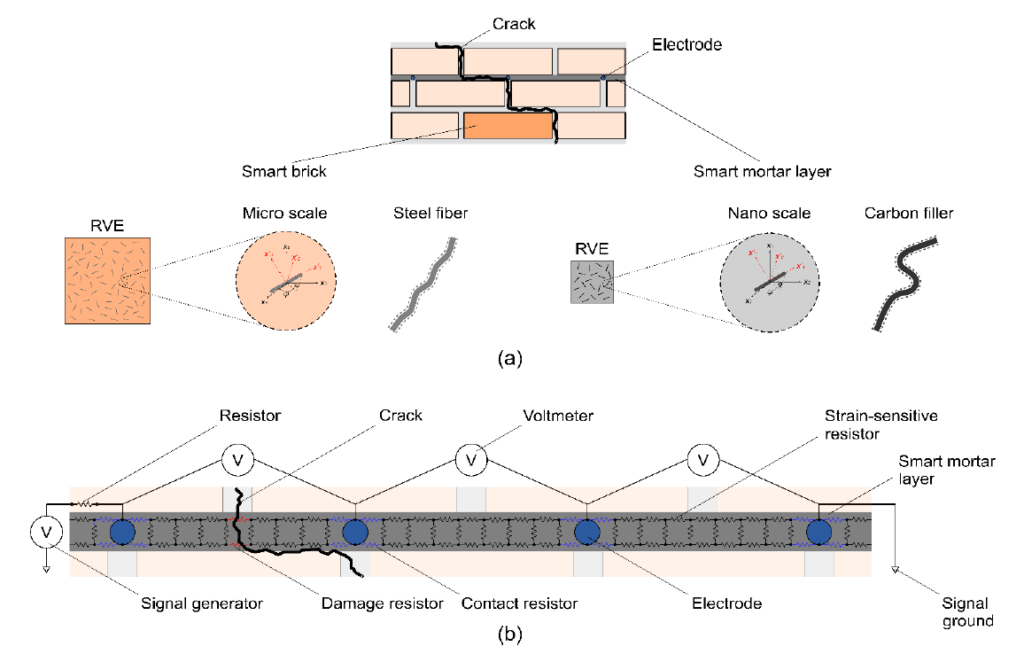
Figure 1. Illustration of proposed modeling approaches for smart masonry based on: (a) a micromechanical approach for smart bricks and (b) a resistor mesh model for smart mortar layer.
Task 2.6 AI-based damage identification algorithms using smart mortars.
Task 2.6 will be a theoretical effort to develop crack detection methods based on machine learning and AI using data outputted by smart mortar layers. Deep learning methods, such as LSTM and autoencoders, will be used to discriminate faulty from sound conditions using reconstruction loss in electromechanical properties of the material as feature. The Smart Masonry enabling SAFEty-assessing STructures after earthquakes (SMS-SAFEST) strain sensing ability of smart mortar will also be exploited for reducing the probability of false positives.
Task 2.7 AI-based damage identification algorithms using smart bricks.
Task 2.7 will be focused on the development of new crack detection methods using smart bricks through advanced data science approaches. Classification features will be based on: (i) deviations of volumetric strain of smart bricks after an earthquake with respect to baseline values, so as to highlight changes in dead loading paths caused by cracks associated to incipient global or local failure mechanisms; (ii) cross-comparisons of the outputs of smart bricks after the earthquake (no baseline needed) so as to evidence regions of strain concentration associated to a structural damage condition.
Task 2.8 SHM strategy using smart masonry.
Task 2.8 will seek for an effective fusion of procedures separately developed within Tasks 2.6 and 2.7 in such a way to define global machine learning and AI-based SHM methods for smart masonry structures under earthquake loading. Information separately provided by smart mortar and smart bricks will be fused to achieve an advanced damage classification, identifying critical issues such as activating failure mechanisms, reached limit states and reduction in residual vertical load bearing capacity of the structure. Key aspects that will also be addressed are: (i) removal of environmental effects through statistical pattern recognition methods and (ii) long-term stability of the classifications.
Task 2.9 Large-scale numerical tests.
Large scale structures with smart bricks and smart mortar layers, representative of real applications, will be modeled. Non-linear static pushover analysis, as well as incremental dynamic analysis will be carried out to simulate the effects of earthquakes of increasing intensities and the possibility to detect a damage at specific limit states of either local or global nature will be verified using the SHM data-science based tools developed within Tasks 2.6-2.8.